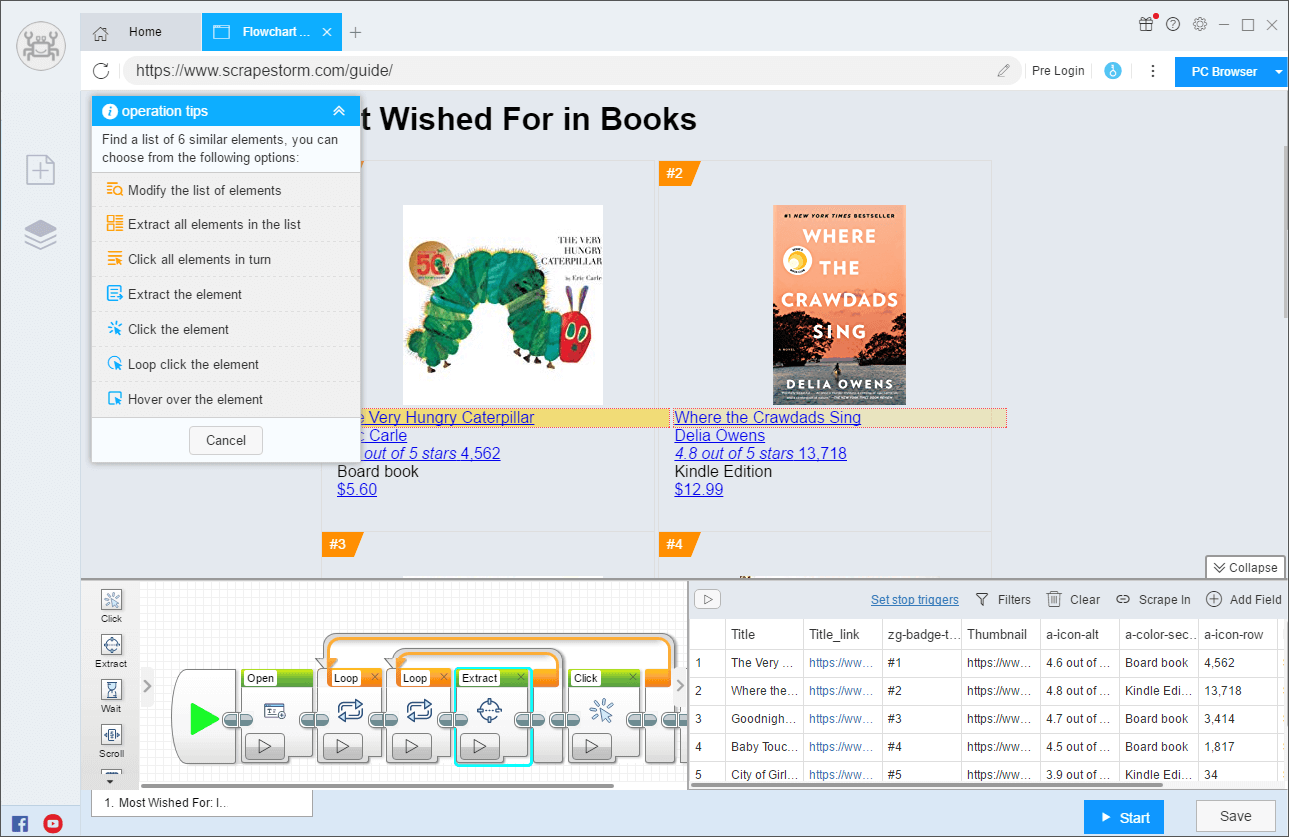
In today’s data-driven world, having access to accurate and timely information is critical for making informed business decisions. However, the process of collecting data from the web can be challenging, especially when dealing with large volumes of information, dynamic websites, or unstructured data. Traditional web scraping techniques, which typically rely on static code Web Scraping Tool and manual adjustments, have become increasingly inadequate for handling modern, complex web pages. Enter AI-powered web scraping, a revolutionary approach that utilizes machine learning, natural language processing (NLP), and other AI technologies to significantly improve the speed, accuracy, and scalability of data collection efforts. By adopting AI-based tools, businesses can boost their data collection efficiency, streamline processes, and gain a competitive edge in an ever-evolving digital landscape.
One of the most notable advantages of AI-driven web scraping is its ability to adapt to dynamic and interactive websites. In the past, scraping tools were limited to extracting data from static HTML pages. However, many modern websites rely on JavaScript, AJAX, and other technologies to load content asynchronously, making it difficult for traditional scraping tools to capture all relevant information. AI-powered scraping tools, however, leverage machine learning algorithms to understand and interact with dynamic content. These tools can detect how data is loaded and predict when and where new content will appear. They can even mimic user behavior by clicking buttons or scrolling through pages to trigger the loading of additional data. As a result, businesses can capture real-time data from highly dynamic websites, ensuring a more complete and accurate collection process.
In addition to handling dynamic websites, AI-powered web scraping enhances the efficiency of data extraction by processing both structured and unstructured data. While structured data, such as tables or product listings, can be easily scraped using traditional methods, much of the valuable information on the web exists in unstructured formats. This includes text, images, videos, and other forms of content that are harder to interpret and analyze. AI technologies like NLP and computer vision enable scraping tools to extract meaning from these unstructured data sources. NLP allows AI to process and understand text, identify sentiment, categorize content, or extract key phrases from large volumes of textual data. Meanwhile, computer vision can be used to analyze images or videos, recognizing objects, reading text within images, or extracting relevant information from visual content. By incorporating these AI capabilities, businesses can collect and analyze a broader range of data, leading to richer insights and more comprehensive intelligence.
Scalability is another area where AI enhances the efficiency of web scraping. As businesses look to gather data from an increasing number of websites or scrape larger datasets, traditional scraping methods can become time-consuming and require constant manual adjustments. Websites frequently change their structure, which means that scraping scripts need to be updated regularly to account for these changes. AI-powered web scraping tools, however, are equipped with self-learning algorithms that continuously adapt to these changes. Machine learning models can automatically analyze new web structures, identify patterns, and adjust the scraping strategy accordingly. This allows businesses to scale their data collection efforts without having to constantly rewrite or update scraping scripts, saving both time and resources. AI tools can scrape data from multiple websites simultaneously, handle vast amounts of data, and scale up or down as needed, ensuring that businesses can meet their data collection demands efficiently.
AI-based web scraping tools also improve the overall quality of the data collected. Traditional scraping methods often result in large volumes of raw data, much of which can be irrelevant, duplicated, or incomplete. This can create additional work, as businesses must spend time cleaning and filtering the data before it can be analyzed. AI-powered scraping tools, on the other hand, automatically prioritize relevant data based on predefined business rules and criteria. Machine learning algorithms can filter out noisy or irrelevant information by learning from previous scraping efforts and refining the data collection process over time. These tools can also detect and correct errors, such as missing data, broken links, or formatting issues, ensuring that only high-quality data is collected. By reducing the need for manual data cleaning and enhancing data accuracy, AI-powered web scraping streamlines the entire data collection process, enabling businesses to focus on analysis and decision-making.
In conclusion, AI-powered web scraping is revolutionizing data collection by enhancing efficiency, scalability, and data quality. By using machine learning, NLP, and computer vision, businesses can capture data from dynamic, interactive websites, process both structured and unstructured content, and scale their scraping operations without constant manual intervention. AI tools are not only faster and more accurate than traditional methods but also help improve the quality of the data collected by filtering out irrelevant or noisy information. As AI technology continues to evolve, it will only continue to transform the web scraping landscape, offering businesses an even more powerful way to gather the data they need to make informed decisions and stay competitive in an increasingly data-centric world. Adopting AI-driven web scraping tools is no longer just an option—it’s a necessity for businesses looking to unlock the full potential of web data and drive success in today’s digital economy.